Can Mixture of Agents (MoA) Beat GPT-4.0?
The Mixture of Agents (MoA) approach excels in scenarios requiring specialized expertise and adaptability, leveraging collaboration among multiple agents. While GPT-4.0 remains a powerful tool for general-purpose natural language processing, MoA has the potential to outperform it in complex, dynamic environments.
Published on:
July 12, 2024
We humans are evolving at a very fast pace and continually strive to discover new things through our experiments and approaches. We always seek to get the advanced version of advanced, perpetually looking for solutions that can be better than the previous ones. This relentless pursuit takes our search journey to the next level.
In recent years, artificial intelligence (AI) has experienced significant advancements, leading to the development of highly sophisticated models capable of performing complex tasks with remarkable accuracy. Among these advancements, OpenAI's Generative Pre-trained Transformer 4.0 (GPT-4.0) stands out as a monumental leap in the realm of natural language processing (NLP).
GPT-4.0 has garnered widespread acclaim for its ability to generate coherent and contextually relevant text, making it a powerful tool for various applications, from automated content creation to customer support.
However, as impressive as GPT-4.0 is, the field of AI continues to evolve, with new paradigms emerging that challenge the dominance of single-model architectures. One such paradigm is the Mixture of Agents (MoA), a concept that leverages multiple specialized agents working collaboratively to achieve a common goal. This approach has shown promise in enhancing decision-making, improving adaptability, and tackling tasks that require diverse expertise.
Let’s explore the potential of Mixture of Agents (MoA) to surpass GPT-4.0 in terms of performance, flexibility, and real-world applicability. We will delve into the key characteristics of both GPT-4.0 and MoA, compare their strengths and weaknesses, and examine the circumstances under which MoA might offer superior solutions.
Understanding GPT-4.O
Overview of the Development of GPT-4o
One of the recent developments that happened in the Artificial Intelligence industry is the unveiling of GPT-4.O, which is an advanced version of GPT-4. This is the fourth version of the generative artificial intelligence pre-trained transformer series, which involves a Large Language Model ( LLM). GPT-4 gained significant popularity among users due to its advanced results.
Whereas the development of GPT-4.O represents a significant milestone in the evolution of language models. Building on the successes of its predecessors, GPT-4o incorporates advancements in deep learning, unsupervised learning, and transformer architectures. These improvements have enabled GPT-4.O to generate human-like text with unprecedented accuracy and coherence.
Key Features and Functionalities of GPT-4.O
Now that you understand GPT-4 and GPT-4.O, you should get more clarity on GPT-4.O. This advanced version offers several features that set it apart from previous models. By collecting feedback from users, making many successful tests helps developers frame new architectures for the latest one. The new version of the GPT model “gets into gear” and provides more explanatory or detailed content than more general prompts. Having said that, the GPT-4.O has a wider word limit, so it can give the desired results in simple input.
Let’s take a closer look at the key features of GPT-4.O
These include:
Enhanced Contextual Understanding:
GPT-4.0 can understand and generate responses based on long conversations, maintaining the context over multiple exchanges. For instance, in a customer support scenario, GPT-4.0 can remember previous interactions and provide consistent and relevant answers based on past conversations.
Example Interaction:
User’s Prompt: "Last week, I contacted you about a refund for my order. Can you update me on the status?"
GPT-4.0: "Sure, I see that your refund request for order #12345 was processed two days ago. You should receive the amount in your account within 3-5 business days."
.png)
Scalability:
GPT-4.0 can handle large-scale data processing tasks efficiently, making it suitable for applications requiring the analysis of vast datasets.
Its scalability can be seen in the field of research, where GPT-4.0 can sift through thousands of academic papers to summarize current trends and emerging topics.
Versatility:
GPT-4.0 is versatile and can be applied to various NLP tasks such as text generation, translation, summarization, and question-answering.
For instance, GPT-4.0 can be employed by a global company to translate business documents from English to multiple languages accurately. Like you can uses GPT-4.0 to translate your annual financial report into 15 different languages, and it will maintain the nuances and accuracy of the original document.
You can also watch:
Use Cases and Applications of GPT-4.O
Let's examine the industry-related use cases to get a clear picture of GPT-4o.
Content Creation
GPT-4.0 can generate high-quality content for blogs, articles, and social media posts. A marketing agency might use GPT-4.o to create engaging blog posts on trending topics, saving time and effort while maintaining a consistent content schedule.
Customer Support
GPT-4.0 can be integrated into customer support systems to provide instant, accurate responses to customer queries. For example, an e-commerce platform might use GPT-4.0 to handle common customer inquiries about order status, returns, and product information.
Example Interaction:
Customer: "Can you tell me when my order #98765 will be delivered?"
GPT-4.0: "Your order #98765 is scheduled for delivery on June 21st. You can track your order using the tracking number provided in your email."
Education
GPT-4.0 can assist in creating educational content and providing tutoring support. An online learning platform could use GPT-4.0 to generate practice questions and explanations for various subjects, helping students learn more effectively.
Healthcare
In healthcare, GPT-4.0 can be used to generate medical reports and summarize patient information. For instance, a hospital might use GPT-4.0 to draft patient discharge summaries based on medical records, ensuring consistency and accuracy.

Limitations and Challenges Faced by GPT-4.0
Despite its impressive capabilities, GPT-4.0 is not without limitations:
- Resource Intensity: Training and deploying GPT-4.0 require substantial computational resources and energy consumption.
- Bias and Fairness: GPT-4.0 can inadvertently perpetuate biases present in the training data, raising ethical concerns.
Context Limitations: While GPT-4.0 has improved contextual understanding, it may still struggle with complex or ambiguous queries that require deep contextual knowledge.
%20(1).png)
Introduction to Mixture of Agents (MoA)
What is the Mixture of Agents (MoA)?
Mixture of Agents (MoA) refers to an AI paradigm that employs multiple specialized agents, each designed to handle specific aspects of a task. These agents work collaboratively, leveraging their individual strengths to achieve a common objective. The MoA approach contrasts with single-model architectures, such as GPT-4.0, by emphasizing the synergy of diverse agents.
The concept of multi-agent systems has been explored in AI research for decades, but recent advancements in machine learning and computational power have made the practical implementation of MoA more feasible. The evolution of MoA has been driven by the need to tackle increasingly complex problems that require diverse expertise and collaborative problem-solving.
Key Components and Architecture of MoA
An MoA system typically comprises:
- Specialized Agents: Individual agents or also considered open-source LLM agents with expertise in specific areas or tasks.
- Coordinator: A central component that manages the interaction and collaboration among agents.
- Communication Protocols: Mechanisms that facilitate information exchange and coordination among agents.
Use Cases and Applications of MoA
The Mixture of Agents (MoA) approach is versatile, like GPT-4o and Attri’s Generative AI Engine, and can be applied across various industries and scenarios. Let’s delve into that, too. Below are some detailed use cases and applications highlighting the effectiveness and benefits of MoA systems.
1. Mixture of Agents(MoA) Use Case for Robotics
Purpose: Search and Rescue Operations
This is not fascinating anymore that Robots are getting involved in our emergency or rescue operations. The best use of Robots can be described if they are used for humanity support. But How?
Multiple robots can be deployed in search and rescue missions. For instance, one robot may be equipped with thermal imaging to locate survivors, another with medical supplies to provide first aid and a third with mapping capabilities to navigate through debris. The central coordinator ensures seamless communication and collaboration among the robots, optimizing the overall mission efficiency.

Image Source: NY Times
Read this BBC Article to learn more about rescue robots using Mixture of Agents (MoA)
2. Mixture of Agents(MoA) Use Case in Healthcare
Purpose: Comprehensive Patient Care
In healthcare, MoA systems can integrate diagnostic agents, treatment planning agents, and monitoring agents. A diagnostic agent can analyze patient data to identify potential health issues, a treatment planning agent can devise personalized treatment plans, and a monitoring agent can track patient progress and adjust the treatment as necessary. The central coordinator facilitates communication between these agents, ensuring holistic and coordinated patient care.
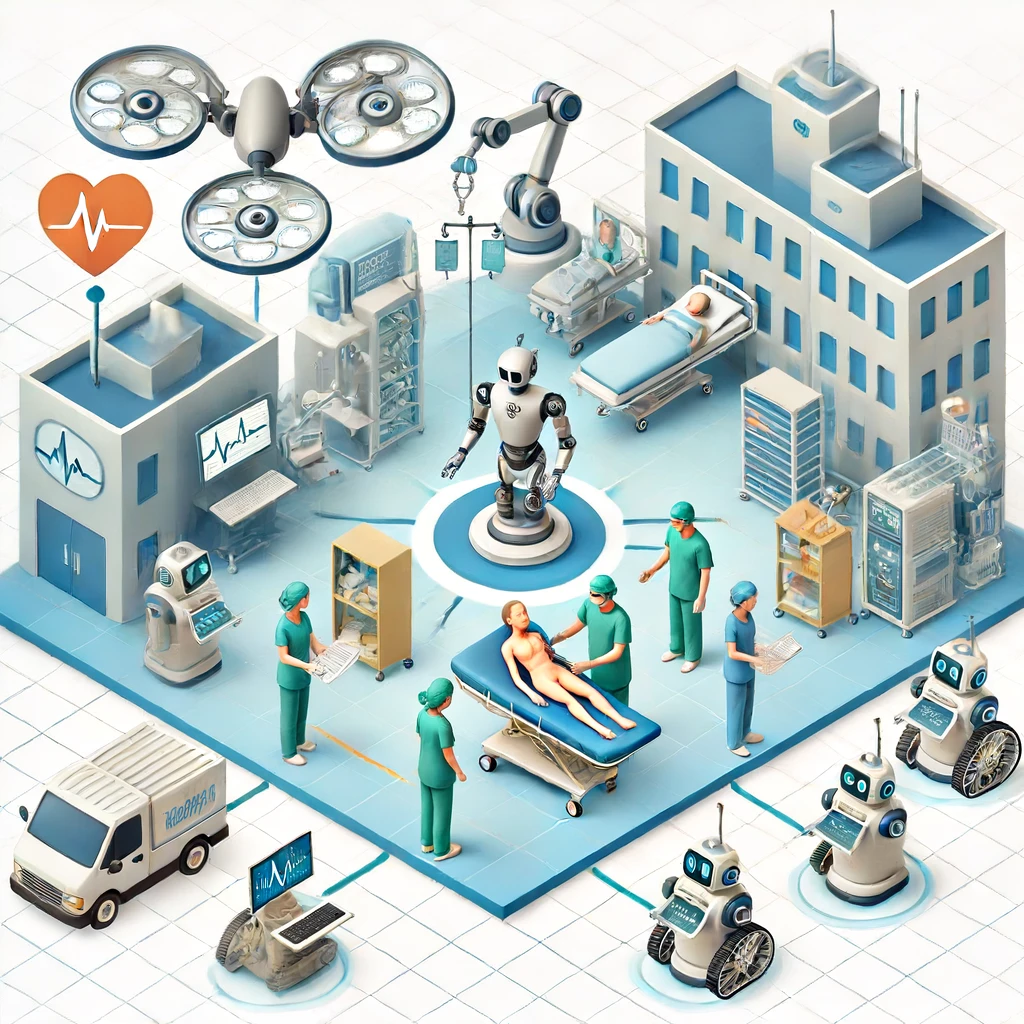
3. Mixture of Agents(MoA) Use Case in Finance
Purpose: Optimizing Investment Strategies
In the financial sector, MoA systems can combine market analysis agents, trading agents, and risk assessment agents. Market analysis agents gather and interpret market data, trading agents execute trades based on this analysis, and risk assessment agents evaluate the potential risks associated with different investment strategies. The central coordinator ensures that these agents work together to optimize investment decisions.

4. Mixture of Agents(MoA) Use Case for Smart Cities
Purpose: Managing Urban Infrastructure and Services
In smart cities, MoA systems can coordinate multiple agents to manage urban infrastructure and services. Traffic management agents can optimize traffic flow, energy distribution agents can balance the supply and demand of energy, and public safety agents can monitor and respond to security incidents. The central coordinator ensures that these agents work harmoniously to improve urban living conditions.
.png)
5. Mixture of Agents(MoA) Use Case for Supply Chain Management
Purpose: Enhancing Logistics and Inventory Management
In supply chain management, MoA systems can integrate logistics agents, inventory management agents, and demand forecasting agents. Logistics agents optimize the transportation of goods, inventory management agents ensure optimal stock levels, and demand forecasting agents predict future demand to adjust procurement strategies. The central coordinator facilitates communication among these agents, ensuring a smooth and efficient supply chain.
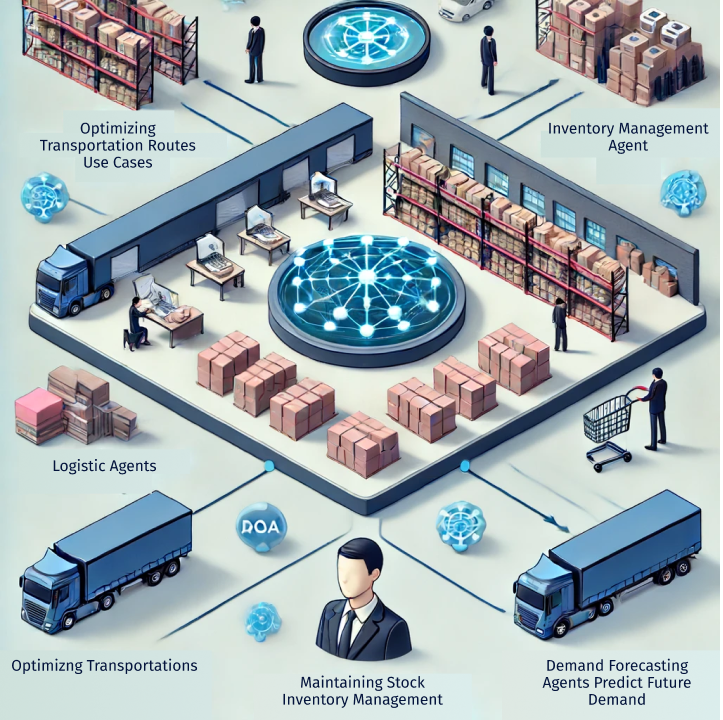
6. Mixture of Agents(MoA) Use Case for Customer Service
Purpose: Providing Comprehensive Customer Support
In customer service, MoA systems can combine query handling agents, troubleshooting agents, and feedback analysis agents. Query handling agents address general customer inquiries, troubleshooting agents resolve specific issues, and feedback analysis agents gather and interpret customer feedback to improve service quality. The central coordinator ensures that customer interactions are handled smoothly and efficiently.
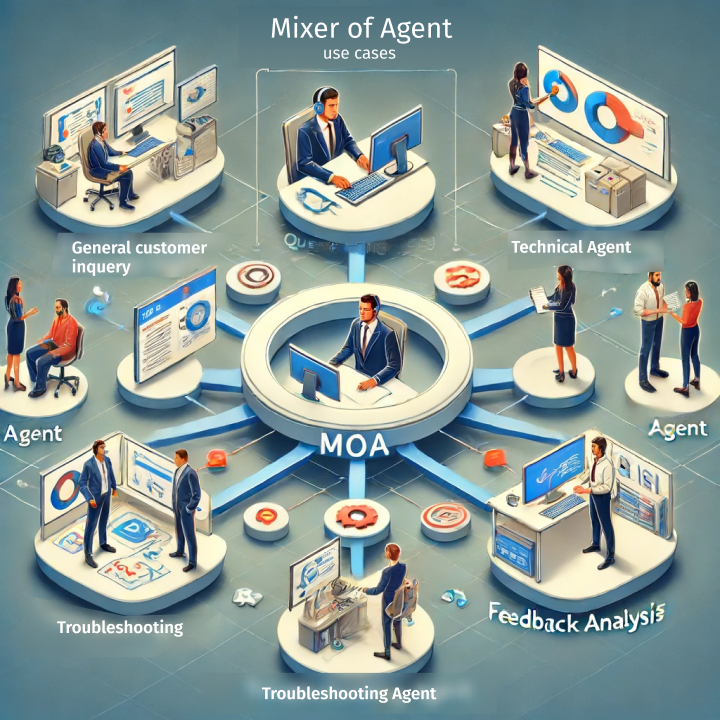
7. Mixture of Agents(MoA) Use Case for Education
Purpose: Personalized Learning Experiences
MoA systems can integrate content delivery agents, assessment agents, and tutoring agents in education. Content delivery agents provide educational materials, assessment agents evaluate student performance, and tutoring agents offer personalized assistance based on individual learning needs. The central coordinator ensures a cohesive and personalized learning experience for students.
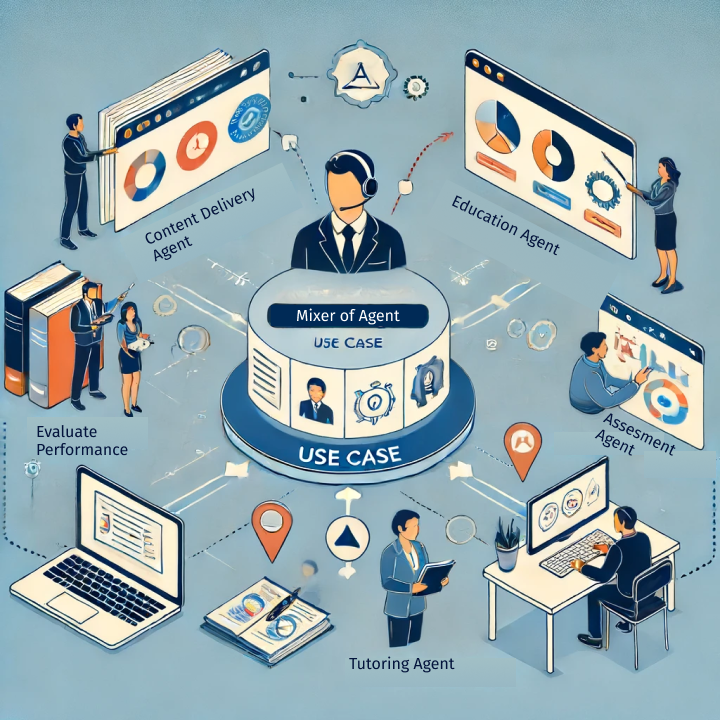
Advantages / Benefits of Mixture of Agents (MoA) Over GPT-4o
Improved Decision-Making and Problem-Solving Capabilities
MoA systems leverage the collective intelligence of multiple agents, leading to more robust and well-rounded decision-making processes. Each agent contributes specialized knowledge, resulting in solutions that are often more accurate and effective than those generated by a single model like GPT-4.0.
Enhanced Collaboration and Coordination Among Agents
The collaborative nature of MoA allows for seamless coordination among agents, enabling them to tackle tasks that require a multifaceted approach. This synergy enhances the system's overall performance, especially in scenarios where diverse expertise is crucial.
Greater Adaptability to Diverse and Dynamic Environments
MoA systems are inherently flexible, as agents can be added, removed, or modified to adapt to changing environments and requirements. This adaptability makes MoA a powerful tool for applications that involve dynamic and unpredictable conditions.
Potential for Continuous Learning and Self-Improvement
Individual agents within an MoA system can be continuously trained and improved, allowing the system to evolve and enhance its capabilities over time. This continuous learning approach ensures that the MoA remains effective and relevant in the face of new challenges.
%20(1).png)
Challenges and Limitations of Mixture of Agents (MoA)
Complexity in Design and Implementation
Designing and implementing an MoA system can be complex, requiring careful planning and coordination. Integrating multiple agents with different expertise and ensuring seamless collaboration presents significant technical challenges.
Scalability Issues
As the number of agents increases, managing their interactions and communications becomes more challenging. Scalability is a critical concern that needs to be addressed to ensure the effectiveness of MoA systems in large-scale applications.
Resource-Intensive Nature
Training and maintaining multiple specialized agents can be resource-intensive, both in terms of computational power and data requirements. Efficient resource management is essential to mitigate these challenges and ensure the practicality of MoA systems.
Ethical and Security Concerns
The collaborative nature of MoA systems raises ethical and security concerns, such as ensuring the fairness and transparency of agents' decisions. Additionally, safeguarding the system against malicious agents or external threats is crucial to maintaining its integrity and reliability.

Can the Mixture of Agents (MoA) Really Outperform GPT-4.0?
Well, the ongoing advancements in artificial intelligence have led to the development of sophisticated models such as OpenAI's GPT-4.0, known for its impressive capabilities in natural language processing.
However, a new paradigm in AI, the Mixture of Agents (MoA), presents a compelling alternative. MoA systems leverage the collaborative power of multiple specialized agents, each optimized for specific tasks coordinated by a central overseer.
We have tried to explain the fundamental differences between GPT-4.0 and MoA, highlighting MoA's advantages in terms of decision-making, adaptability, efficiency, and scalability across various real-world applications such as healthcare, finance, robotics, smart cities, supply chain management, customer service, and education.
From the above explanation, it is evident that Mixture of Agents(MoA) can have more potential than GPT-4.o. By comparing these two AI approaches, we can understand that MoA could potentially surpass GPT-4.0 in performance and applicability.
Conclusion
In conclusion, the Mixture of Agents (MoA) approach offers several distinct advantages over GPT-4.0. MoA systems excel in environments that require specialized expertise, dynamic adaptability, and collaborative problem-solving.
The ability of MoA to integrate and coordinate multiple agents with distinct roles allows it to handle complex and multifaceted tasks more effectively than a single, generalized model like GPT-4.0. Furthermore, MoA's scalability and potential for continuous improvement make it a robust and future-proof solution for various industries.
While GPT-4.0 remains a powerful and versatile model, its limitations in handling highly specialized tasks, resource intensity, and potential bias issues highlight the benefits of adopting an MoA approach in certain contexts. The real answer to whether MoA can beat GPT-4.0 depends on the specific application and requirements.
In scenarios where collaboration, specialization, and adaptability are crucial, MoA can outperform GPT-4.0. Therefore, while GPT-4.0 continues to be a significant achievement in AI, the Mixture of Agents approach represents a promising direction for the future of artificial intelligence, offering capabilities that can complement and, in some cases, surpass those of GPT-4.0.
At Attri, we have leveraged the power of agents that you can explore among our Generative AI solutions.