The Ultimate Guide to Predictive Maintenance
In our comprehensive guide to predictive maintenance, you'll learn everything you need to know to optimize your maintenance strategy. From understanding the key components to reaping the benefits and implementing best practices, this guide equips you with the knowledge to revolutionize your operations and maximize equipment uptime.
Published on:
April 29, 2024
In today's rapidly evolving industrial landscape, predictive maintenance (PM) has emerged as a game-changer for businesses across various sectors. By harnessing advanced technologies and data analytics, predictive maintenance enables organizations to optimize equipment performance, minimize downtime, and reduce operational costs.
This comprehensive guide delves into the intricacies of predictive maintenance, exploring its definition, significance, and key components.
At its core, predictive maintenance (PM) is a proactive approach to maintenance Management that leverages data analytics, machine learning, and sensor technology to predict equipment failures before they occur.
Unlike traditional maintenance strategies such as reactive maintenance (fixing equipment after it breaks down) and preventive maintenance (conducting routine maintenance at predetermined intervals), predictive maintenance relies on real-time data and predictive algorithms to identify potential issues and schedule maintenance activities accordingly.
Importance of Predictive Maintenance in Various Industries
Predictive maintenance holds immense significance across a wide range of industries, from manufacturing and energy to healthcare and transportation. In manufacturing, for example, the ability to predict equipment failures enables companies to avoid costly unplanned downtime and optimize production schedules.
Similarly, in the energy sector, predictive maintenance plays a crucial role in ensuring reliability and efficiency of critical infrastructure such as wind turbines and power plants. Moreover, in healthcare settings, predictive maintenance helps hospitals maintain the uptime of medical equipment, ensuring uninterrupted patient care and treatment.
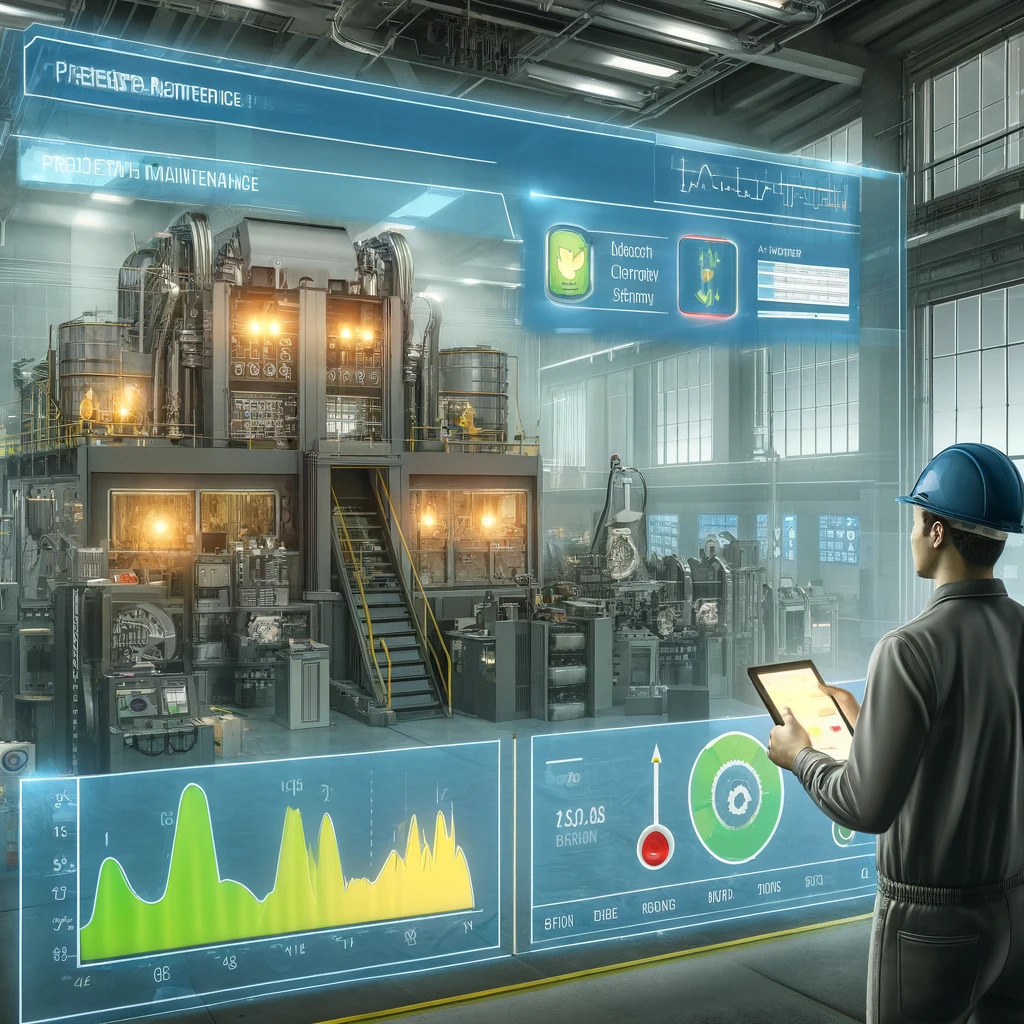
Understanding Predictive Maintenance
Explanation of Predictive Maintenance Concept
Predictive maintenance operates on the principle of proactive intervention based on data-driven insights. Predictive maintenance systems can detect anomalies and patterns indicative of potential failures by continuously monitoring equipment performance and collecting data on factors such as temperature, vibration, and energy consumption.
This proactive approach allows organizations to address issues before they escalate into costly breakdowns, thereby maximizing equipment uptime and productivity.
Comparison with Other Maintenance Strategies
While traditional maintenance strategies such as reactive maintenance and preventive maintenance have their merits, they often fall short in terms of cost-effectiveness and reliability.
Reactive maintenance, for instance, is inherently reactive in nature, relying on equipment failures to trigger maintenance actions. This approach can lead to unplanned downtime, increased repair costs, and compromised safety.
Preventive maintenance, on the other hand, involves performing routine maintenance tasks at fixed intervals, regardless of the actual condition of the equipment. While preventive maintenance can help prevent some failures, it may also result in unnecessary maintenance activities and associated costs.
In contrast, predictive maintenance takes a proactive and data-driven approach to maintenance management. By analyzing real-time data and using advanced algorithms to predict equipment failures, predictive maintenance enables organizations to optimize maintenance schedules, minimize downtime, and reduce maintenance costs. Additionally, predictive maintenance helps extend the lifespan of equipment, improve safety, and enhance overall operational efficiency.
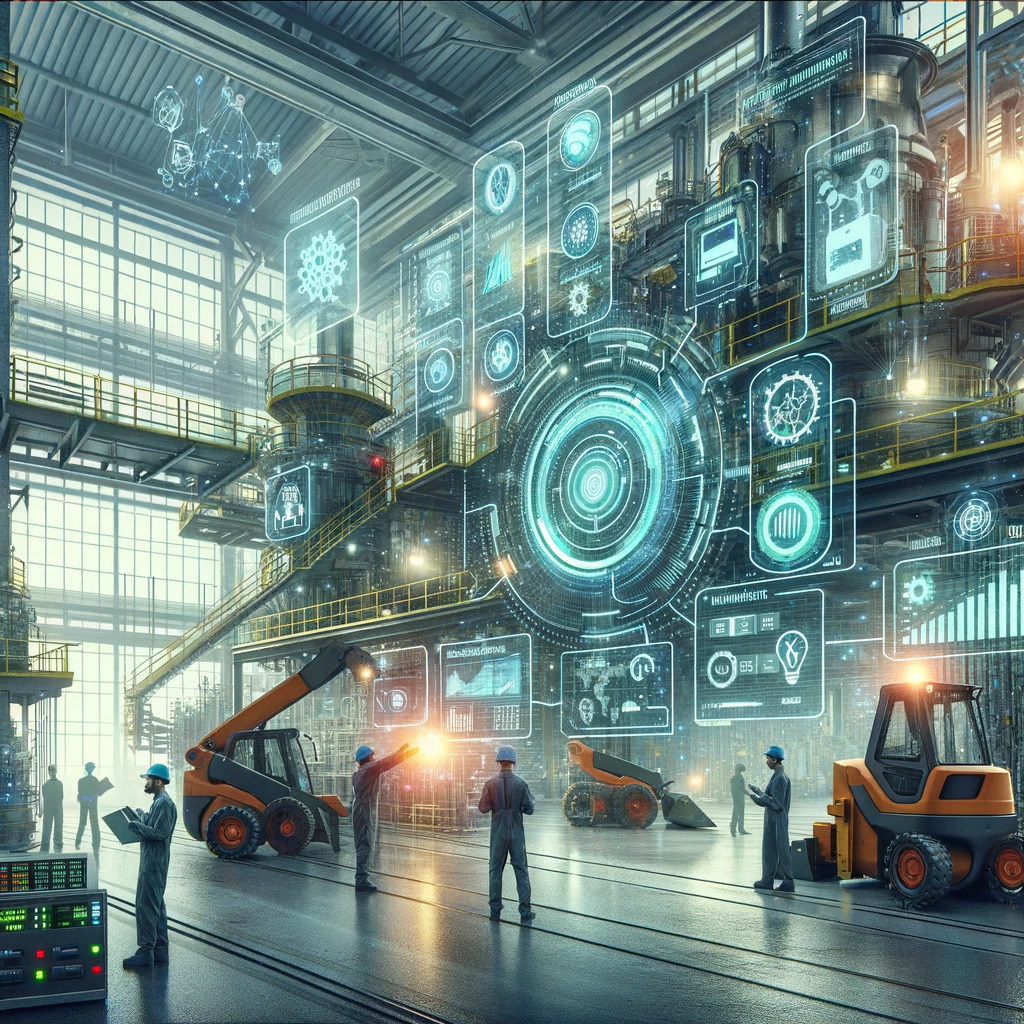
Learn more about the critical elements of predictive maintenance.
Key Components of Predictive Maintenance
Data Collection Techniques
One of the cornerstones of predictive maintenance is the use of advanced generative AI data collection techniques to gather real-time information about equipment performance. Sensor-based monitoring is a primary method employed in predictive maintenance systems.
These sensors are strategically placed on machinery and equipment to continuously monitor parameters such as temperature, vibration, pressure, and energy consumption.
The data collected by these sensors provide valuable insights into the health and condition of the equipment, allowing maintenance teams to identify potential issues early on.
Data Analysis Methods
Once the data is collected, sophisticated data analysis methods are employed to extract actionable insights and predict equipment failures. Trend analysis is a common technique used to identify patterns and anomalies in the data that may indicate impending failures.
By analyzing historical data and comparing it with real-time measurements, predictive algorithms can detect deviations from normal operating conditions and predict when equipment is likely to fail.
Additionally, fault diagnosis algorithms are used to pinpoint the root causes of failures and identify the most appropriate course of action for maintenance.
Implementation Strategies
Implementing predictive maintenance requires careful planning and integration with existing systems and processes. Organizations need to invest in the right technologies and tools to collect and analyze data effectively. This may involve deploying sensor networks, investing in predictive maintenance software, and training personnel on how to use these tools.
Additionally, organizations must develop maintenance strategies that align with predictive maintenance principles, such as condition-based maintenance and reliability-centered maintenance. By integrating predictive maintenance into their operations, organizations can realize significant benefits in terms of equipment reliability, uptime, and cost savings.
Let’s talk about your strategies.
Industries Benefiting from Predictive Maintenance
1. Manufacturing
The manufacturing industry is one of the primary beneficiaries of predictive maintenance. By implementing predictive maintenance strategies, manufacturers can optimize production schedules, reduce unplanned downtime, and improve overall equipment effectiveness (OEE).
For example, a manufacturer may use predictive maintenance to monitor the performance of critical machinery such as CNC machines and robots. By analyzing data from sensors installed on these machines, maintenance teams can detect early signs of wear and tear and schedule maintenance activities proactively, thereby minimizing disruptions to production processes and maximizing efficiency.
2. Energy Sector
In the energy sector, predictive maintenance plays a crucial role in ensuring the reliability and efficiency of critical infrastructure such as wind turbines, power plants, and transmission lines.
By continuously monitoring equipment performance and predicting potential failures, energy companies can prevent costly downtime, optimize energy production, and improve asset reliability. For example, a wind farm operator may use predictive maintenance to monitor the health of wind turbine components such as gearboxes and blades.
By detecting signs of fatigue or damage early on, maintenance teams can schedule repairs proactively, avoiding catastrophic failures that could disrupt power generation and pose risks to personnel.
3. Healthcare
The healthcare industry relies heavily on medical equipment to deliver quality patient care and treatment. Predictive maintenance plays a vital role in ensuring the uptime and reliability of critical medical equipment such as MRI machines, CT scanners, and X-ray machines.
By monitoring the performance of these devices in real-time and predicting potential malfunctions, healthcare organizations can minimize downtime, reduce repair costs, and ensure uninterrupted patient care.
For example, a hospital may use predictive maintenance to monitor the condition of MRI machine components such as coils and magnets. By analyzing data from sensors installed on these components, maintenance teams can detect early signs of deterioration and schedule maintenance activities proactively, ensuring that the equipment is always available when needed for patient examinations and procedures.
Challenges and Solutions
Data Quality and Integration
One of the primary challenges organizations face when implementing predictive maintenance is ensuring the quality and integration of data from various sources. Data may often be fragmented or siloed across different systems, making accessing and analyzing difficult.
To address this challenge, organizations need to invest in robust data integration solutions that allow for seamless aggregation and analysis of data from sensors, equipment, and other sources.
Additionally, ensuring the accuracy and reliability of data is crucial for making informed maintenance decisions. This may involve implementing data validation and cleansing processes to identify and correct any inaccuracies or inconsistencies in the data.
Skilled Workforce and Training
Another challenge organizations face is building and retaining a skilled workforce capable of implementing and managing predictive maintenance systems. Predictive maintenance requires specialized knowledge and expertise in areas such as data analytics, machine learning, and sensor technology.
To overcome this challenge, organizations need to invest in training and education programs to upskill existing employees and attract new talent. This may involve providing hands-on training on predictive maintenance tools and techniques and offering certification programs to validate employees' skills and knowledge.
Cost of Implementation
Implementing predictive maintenance systems can be costly and resource-intensive, requiring significant investments in technology, infrastructure, and personnel. For many organizations, the upfront costs of implementing predictive maintenance may be prohibitive, particularly for small and medium-sized enterprises (SMEs) with limited budgets.
To address this challenge, organizations need to carefully evaluate the return on investment (ROI) of predictive maintenance and develop a comprehensive business case that demonstrates the potential cost savings and benefits.
Additionally, exploring alternative funding options such as grants, subsidies, or financing programs can help offset the initial costs of implementation and make predictive maintenance more accessible to a wider range of organizations.
Overcoming Challenges with Innovative Solutions
While the challenges of implementing predictive maintenance may seem daunting, organizations can overcome them with innovative solutions and strategies. For example, leveraging cloud-based predictive maintenance platforms can provide organizations with scalable, cost-effective solutions that require minimal upfront investment in infrastructure.
Additionally, partnering with third-party vendors and service providers can help organizations access specialized expertise and resources that may not be available in-house. By embracing innovation and exploring creative solutions, organizations can overcome the challenges of implementing predictive maintenance and unlock the full potential of this powerful technology.
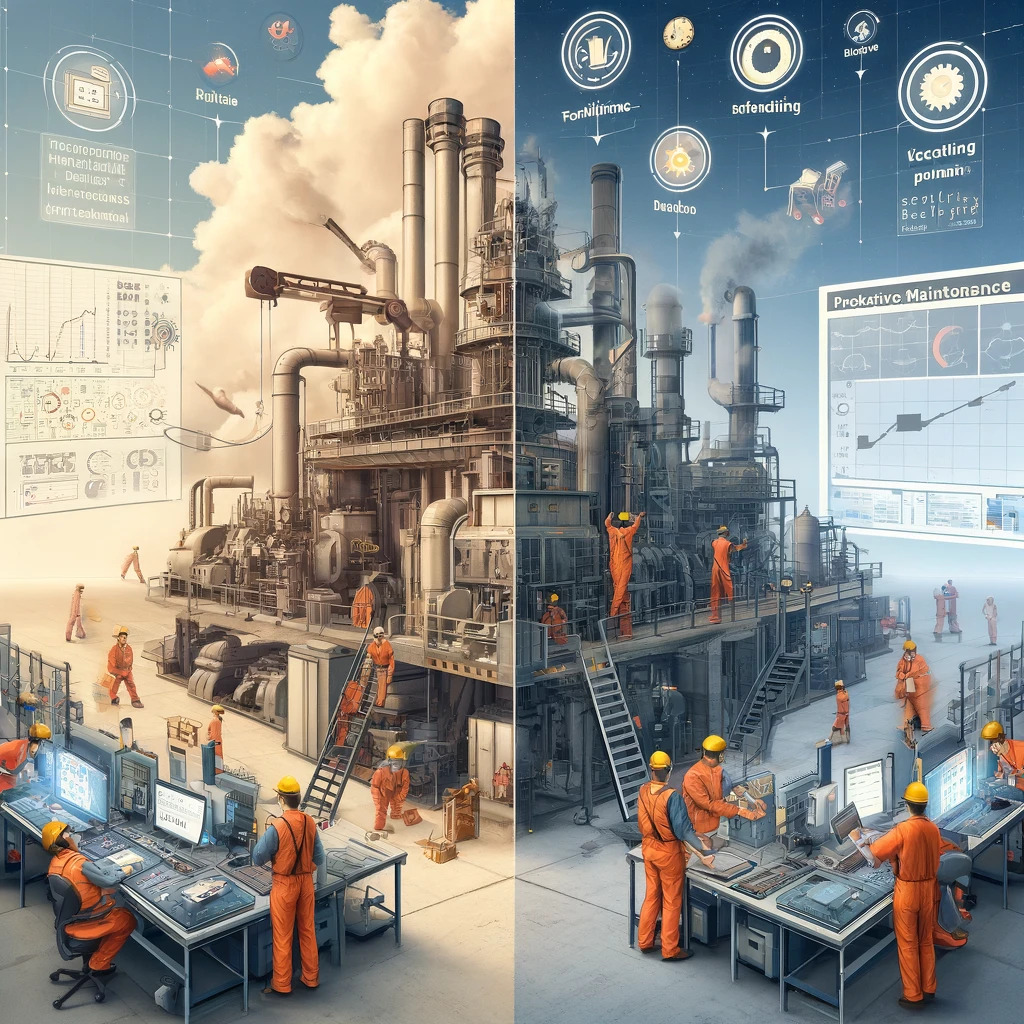
Future Trends and Technologies
1. Internet of Things (IoT) in Predictive Maintenance
The Internet of Things (IoT) is poised to play a significant role in the future of predictive maintenance. IoT devices such as sensors, actuators, and connected machines enable organizations to collect vast amounts of data in real-time from across their operations.
By harnessing this data and leveraging advanced analytics techniques, organizations can gain deeper insights into equipment performance, identify emerging issues, and predict failures before they occur. Additionally, IoT-enabled predictive maintenance systems can enable remote monitoring and diagnostics, allowing maintenance teams to access critical information and perform maintenance activities from anywhere, at any time.
2. Predictive Analytics Advancements
in predictive analytics drive innovation in predictive maintenance, enabling organizations to extract deeper insights from their data and make more accurate predictions about equipment performance and reliability.
Machine learning algorithms, in particular, are becoming increasingly sophisticated, allowing organizations to identify complex patterns and correlations in their data that may not be apparent to human analysts.
By combining predictive analytics with other emerging technologies such as, artificial intelligence and big data, organizations can develop more robust and reliable predictive maintenance models that can adapt and evolve over time.
3. Artificial Intelligence and Machine Learning Applications
Artificial intelligence (AI) and machine learning (ML) are revolutionizing predictive maintenance by enabling organizations to analyze vast amounts of data and make informed decisions in real-time. AI and ML algorithms can identify hidden patterns and trends in data, predict equipment failures with greater accuracy, and recommend optimal maintenance strategies.
For example, AI-powered predictive maintenance systems can automatically generate maintenance schedules, prioritize maintenance tasks based on risk and criticality, and optimize resource allocation to minimize costs and maximize efficiency. As AI and ML technologies continue to evolve, organizations can expect to see even greater advancements in predictive maintenance capabilities, enabling them to achieve new levels of efficiency, reliability, and competitiveness.
Conclusion
In conclusion, predictive maintenance represents a paradigm shift in maintenance management, offering organizations a proactive and data-driven approach to optimizing equipment performance and minimizing downtime. By leveraging advanced technologies such as sensor-based monitoring, data analytics, and machine learning, organizations can predict equipment failures before they occur, enabling them to schedule maintenance activities proactively, reduce unplanned downtime, and optimize operational efficiency.
As we've explored in this guide, predictive maintenance has significant implications across various industries, from manufacturing and energy to healthcare and transportation. By implementing predictive maintenance strategies, organizations can improve equipment reliability, extend asset lifespan, and reduce maintenance costs. Additionally, predictive maintenance helps organizations enhance safety, minimize environmental impact, and achieve regulatory compliance.
While the challenges of implementing predictive maintenance may be daunting, organizations can overcome them by embracing innovation, investing in training and education, and exploring alternative funding options. By doing so, organizations can unlock the full potential of predictive maintenance and reap the benefits of improved reliability, efficiency, and competitiveness.
If you have any further questions or would like to learn more about predictive maintenance, please get in touch with us.